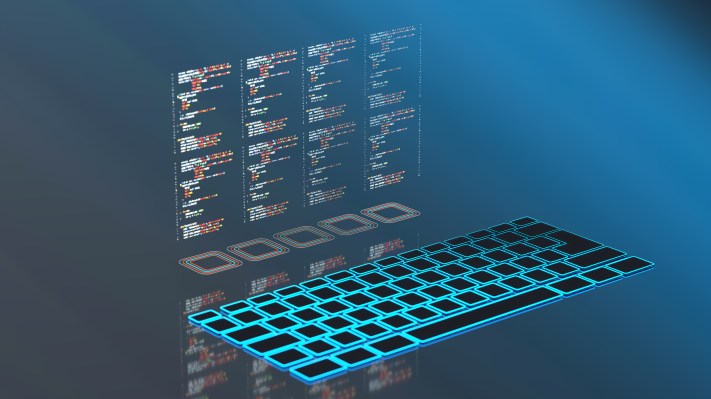
Of all enterprise departments, product and engineering spend by far essentially the most on AI know-how. Doing so successfully stands to generate large worth — builders can full sure duties as much as 50% quicker with generative AI, in line with McKinsey.
However that’s not as straightforward as simply throwing cash at AI and hoping for the very best. Enterprises want to know how a lot to finances into AI instruments, weigh the advantages of AI versus new recruits, and the way to make sure their coaching is on level. A latest research additionally discovered that who is utilizing AI instruments is a essential enterprise resolution, as much less skilled builders get much more advantages out of AI than skilled ones.
Not making these calculations might result in lackluster initiatives, a wasted finances and even a lack of workers.
At Waydev, we’ve spent the previous 12 months experimenting on one of the simplest ways to make use of generative AI in our personal software program improvement processes, creating AI merchandise, and measuring the success of AI instruments in software program groups. That is what we’ve discovered on how enterprises want to arrange for a severe AI funding in software program improvement.
Perform a proof of idea
Many AI instruments rising at present for engineering groups are based mostly on utterly new know-how, so you will have to do a lot of the combination, onboarding and coaching work in-house.
When your CIO is deciding whether or not to spend your finances on extra hires or on AI improvement instruments, you first want to hold out a proof of idea. Our enterprise clients who’re including AI instruments to their engineering groups are doing a proof of idea to determine whether or not the AI is producing tangible worth — and the way a lot. This step is vital not solely in justifying finances allocation but additionally in selling acceptance throughout the crew.
Step one is to specify what you’re trying to enhance throughout the engineering crew. Is it code safety, velocity, or developer well-being? Then use an engineering administration platform (EMP) or software program engineering intelligence platform (SEIP) to trace whether or not your adoption of AI is transferring the needle on these variables. The metrics can range: You could be monitoring velocity utilizing cycle time, dash time or the planned-to-done ratio. Did the variety of failures or incidents lower? Has developer expertise been bettering? All the time embody worth monitoring metrics to make sure that requirements aren’t dropping.
Ensure you’re assessing outcomes throughout a wide range of duties. Don’t limit the proof of idea to a particular coding stage or undertaking; use it throughout various capabilities to see the AI instruments carry out higher beneath totally different situations and with coders of various abilities and job roles.