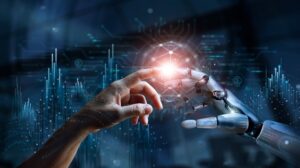
(PopTika/Shutterstock)
The extent of hype round generative AI is off the charts, as now we have coated right here in Datanami over the previous 12 months. The hype is so thick at occasions, you would minimize it with a knife. And but, there’s nonetheless the potential that folks might be underestimating the impression that GenAI can have on enterprise. At the least that’s what the heads of two GenAI software program corporations are saying.
Because the President and Co-founder of Moveworks, Varun Singh has a chook’s eye view of how massive language fashions (LLMs) are impacting the enterprise. The corporate develops a platform that permits clients to leverage GenAI tech to construct chatbots and different kinds of purposes. The corporate counts greater than 100 Fortune 500 corporations as clients.
Whereas the GenAI subject is shifting quick, Singh doesn’t assume folks have bitten off greater than they will chew. “I haven’t seen folks attempting to do an excessive amount of, when it comes to what’s anticipated of them,” Singh says. “To date, what we’re seeing is… individuals are nonetheless coming to phrases with how highly effective these fashions are.”
Moveworks Copilot automates enterprise processes throughout purposes
Moveworks makes use of LLMs like GPT-4 to create chatbots, equivalent to an HR chatbot that solutions questions on firm advantages, or an IT service desk chatbot that may reply questions on IT issues. Extra not too long ago, the corporate has been shifting up the GenAI ladder by serving to clients create GenAI co-pilots that may deal with extra superior duties.
How nicely these GenAI co-pilots are working has been an actual eye-opener for Singh, who anticipates a whole lot of progress on this space in a brief period of time.
“I believe proper now individuals are nonetheless desirous about LLMs as working as brokers, however inside utility boundaries,” he tells Datanami in a current interview. “The following degree of use instances which might be rising, that now we have been doing for some time now, particularly with our subsequent era Moveworks Copilot, is performing as brokers throughout utility boundaries, the place you don’t need to even point out the agent expertise.”
One Moveworks’ Copilot utility was in a position to deal with the duties of 36 completely different human brokers, Singh says. Supplied with the right plug-in to enterprise purposes or knowledge sources (Moveworks has greater than 100 of them), the co-pilot is ready to get entry to the appliance, monitor how human brokers work together with the app, after which recreate the duties by itself.
“It’s utterly insane when it comes to its capability to discern and do actions throughout vary of various purposes and auto deciding on the fitting plugins,” Singh says. “It’s working. And albeit, I don’t assume that’s an excessive amount of at this stage, when it comes to how far you may push this expertise.”
Moveworks will get down into the weeds with GenAI so its clients don’t need to. Its engineers poke and prod the assorted LLMs obtainable on the enterprise market and from open supply repositories to see the place they’ll be a very good match for its clients. “We use GPT-4, however we additionally develop our personal fashions,” Singh says. “We’re experimenting with Llama2. We’re high-quality tuning T5 and different open supply fashions.”
GPT-4, for instance, demonstrates large functionality in language understanding and era, however it will possibly improve latency and has questions round accuracy, so Moveworks makes use of its personal fashions in some conditions, Singh says. Every GenAI deployment usually includes a number of fashions, which Moveworks coordinates behind the scenes.
“Crucial factor for patrons is time to worth, and the price of attending to that worth,” Singh says. “They don’t care if it was GPT3 or 4, or so long as the worker expertise and the outcomes [are there]. And the outcomes they’re searching for is full automation of the service desk.”
The potential proven by GenAI is huge, however we’re not even scratching the floor of what it’s absolutely able to, Singh says.
“These fashions are very highly effective, however we’re not good considering deep sufficient in regards to the utility of those fashions,” he says. “So the disaster is slightly bit on the creativity entrance.”
Are We Underselling GenAI?
Arvind Jain, the CEO and founding father of Glean, has an identical story to inform.
Jain based Glean in 2019 to create customized information bases that enterprises may search to reply questions. The previous Google engineer began working with early language fashions, like Google’s BERT, to deal with the semantic matching of search phrases to enterprise lingo. As LLMs acquired greater, the aptitude of the chatbots acquired even higher.
Glean serves as a ChatGPT-like service for an enterprise
“We really feel that GenAI’s potential is even bigger,” Jain says. “There’s large hype and there’s been some disappointments. However I believe proper now, given how folks really feel, I believe the impression of GenAI is definitely bigger than what most individuals assume in the long term.”
Jain explains that the explanation for his GenAI optimism is how a lot better the expertise has gotten in simply the previous 5 years. Because the expertise improves, it lowers the barrier to entry for individuals who can partake of GenAI, whereas concurrently elevating the standard of what may be constructed.
“5 years again, it was solely corporations like us who may truly use these fashions,” Jain says. “You needed to even have engineering groups. The fashions weren’t as end-user prepared. They have been type of clunky applied sciences, troublesome to make use of, that don’t work that nicely. So then you definately want engineers to do a whole lot of work to tune these fashions and make it work to your use instances.
“However that modified,” he continues. “Now massive language fashions have come to a spot the place it’s gotten democratized in some sense. Now all people within the firm can truly remedy knowledge enterprise issues utilizing these fashions.”
If you wish to construct your individual GenAI chatbot from scratch, it nonetheless takes engineering expertise, Jain says, though anyone with the abilities of a knowledge scientist ought to be capable to put it collectively. And if you wish to construct your individual LLM mannequin–nicely, that piece of tech is basically off the desk for the overwhelming majority of corporations, as a result of immense technical ability required, along with large mounds of coaching knowledge and GPUs to coach them.
However now that very highly effective LLMs are available, engineering outfits like Glean can use them to construct shrink-wrapped GenAI purposes which might be prepared for enterprise on day one. The core Glean providing is principally “like Google and ChatGPT inside your organization,” Jain says. The corporate, which has 200 paying clients, additionally presents a low-code app builder that permits non-technical personnel to construct their very own GenAI apps.
“Firms ought to consider AI as a expertise that they will use, that they will purchase, that they will incorporate into their enterprise processes, into their merchandise with out having to fret about ‘Hey, do I have to construct expertise to begin constructing fashions,’” Jain says. “Only a few corporations want to truly construct and practice fashions.”
For each OpenAI, Google, or Meta that builds their very own LLM from scratch, there can be many extra corporations like Glean that rent engineers and use the LLMs to construct AI merchandise that enterprises will use, Jain says. Nonetheless, a handful of enormous enterprises might resolve that they should construct their very own GenAI merchandise. These enterprises will want engineering expertise.
“Relying on the context, it’s going to require you to have a engineering workforce that’s going to have the ability to successfully use these massive language mannequin expertise and a few RAG-based platform like Glean,” he says. “You would want some engineering to truly incorporate GenAI applied sciences into your enterprise processes and merchandise.
“Then there are additionally going to be many conditions the place you may simply go purchase a product,” he says. “And for that, you should utilize the HR workforce. You don’t have to construct an engineering workforce. You possibly can simply purchase a product like Glean or like many different merchandise like that and simply deploy that and get the worth of AI.”
The longer term is broad open for GenAI, Jain says, notably for corporations who will leverage the expertise to construct compelling new merchandise. We’re simply at first of that transformation, he says. The early returns on GenAI funding are superb already, and the long run is broad open.
“I truthfully really feel just like the expertise is continuous to shock folks. It’s shifting quick. And we’re getting actual worth from it,” Jain says. “The purposes go nicely past chatbot use case. This expertise is sort of broad.”
Associated Objects:
GenAI Hype Bubble Refuses to Pop
Massive Language Fashions: Don’t Imagine the Hype
Massive Language Fashions in 2023: Well worth the Hype?