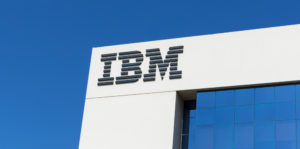
(Laborant/Shutterstock)
Deep studying AI fashions, equivalent to GenAI chatbots, possess an insatiable urge for food for information. These fashions want information for coaching functions to allow them to be efficient for real-world situations.
It may be difficult, by way of effort, compliance, and price, to supply AI fashions with this huge quantity of knowledge and to make sure high quality, relevance, and variety of knowledge. What if we may feed AI fashions with artificial information for coaching functions?
That’s precisely what IBM plans on doing. The tech large desires to make use of artificial information to feed AI’s large urge for food. It’s in search of to patent a system for “artificial information technology” the place it creates a simulation of genuine information from actual customers. It’s going to deploy an modern methodology, known as Giant-Scale Alignment for Chatbots (LAB), which is able to systematically generate artificial information for the duties that builders need their chatbot to perform.
The effectiveness of the AI mannequin is closely reliant on the info it’s skilled on. IBM realized that one of many bottlenecks for speedy AI improvement is the necessity for correct and consultant information for coaching fashions.
Coaching fashions will be expensive and time-consuming, and may usually require devoted sources. The LAB methodology can drastically decrease prices and the time sometimes related to coaching LLMs. It does this by frequently assimilating new data and capabilities into the mannequin with out overwriting what the mannequin already discovered. This could create an abundance of fresh and processed information to coach the AI fashions.
The brand new information technology methodology is predicated on taxonomy – classification of knowledge into classes and subcategories. IBM’s taxonomy works by segregating instruction information into three overarching classes: data, foundational abilities, and compositional abilities.
The taxonomy maps out current abilities and data of the chatbot and highlights gaps that must be stuffed. This technique allows LLM builders to specify desired data and abilities for his or her chatbots.
A second LLM, known as a trainer mannequin, formulates directions based mostly on a question-answer framework tailor-made to the duty. The trainer mannequin goals to additional refine the simulation by producing directions for every class whereas sustaining high quality management. This graduated coaching strategy allows the AI mannequin to progressively construct upon its current data base, much like human studying development.
“Instruction information is the lever for constructing a chatbot that behaves the best way you need it to,” mentioned Akash Srivastava, chief architect of LLM alignment at IBM Analysis. “Our methodology means that you can write a recipe for the issues you need your chatbot to resolve and to generate instruction information to construct that chatbot.”
One of many key advantages of utilizing artificial information is the added privateness. Utilizing actual information for coaching has the inherent danger of spitting that precise private information again out if prompted in a particular approach. With artificial information, you may mirror actual human behaviors, interactions, and decisions, with out violating person privateness.
Whereas artificial information for AI fashions presents a number of advantages, it comes with its personal set of dangers. When you need the artificial information to carefully mimic human habits, if it really mimics an precise person’s information too carefully, then it may very well be an issue, particularly in industries like healthcare and finance.
To check the LAB methodology, IBM Analysis generated an artificial dataset with 1.2 million directions and used that information to coach two open-source LLMs. The outcomes present that each LLMs carried out on par or higher with the state-of-the-art chatbots on a variety of benchmarks. IBM additionally used the artificial information to enhance its personal enterprise-focused Granite fashions on IBM watsonx.
Based on IBM, two distinguishing traits contributed to those spectacular outcomes. Firstly, it’s the means of the trainer mannequin to generate artificial examples from every leaf node of the taxonomy, permitting for broader protection of goal duties.
Secondly, the LAB methodology permits new abilities and data to be added to the bottom LLM with out having to include this data into the trainer mannequin as nicely. “This implies you don’t want some omnipotent trainer mannequin that distills its capabilities into the bottom mannequin,” mentioned David Cox, vp for AI fashions at IBM Analysis.
IBM’s patent additionally highlights that there may very well be an increase in demand for AI providers, and it may very well be simply as profitable as constructing AI itself. It received’t be shocking if IBM makes use of this patent to assist enterprises which might be constructing their very own AI fashions, providing a much less resource-intensive methodology in comparison with amassing genuine person information.
Associated Objects
Why A Dangerous LLM Is Worse Than No LLM At All
The Human Contact in LLMs and GenAI: Shaping the Way forward for AI Interplay
Past the Moat: Highly effective Open-Supply AI Fashions Simply There for the Taking