Introduction
Textual content embedding performs an important position in fashionable AI workloads, significantly within the context of enterprise search and retrieval programs. The flexibility to precisely and effectively discover probably the most related content material is prime to the success of AI programs. Nonetheless, present options for textual content embedding have sure limitations that hinder their effectiveness. Snowflake, a outstanding participant in AI expertise, has just lately developed an open-source resolution revolutionizing textual content embedding duties. The Snowflake Arctic embed household of fashions offers organizations with cutting-edge retrieval capabilities, particularly in Retrieval Augmented Era (RAG) duties. Let’s delve into the main points of those new textual content embedding fashions.
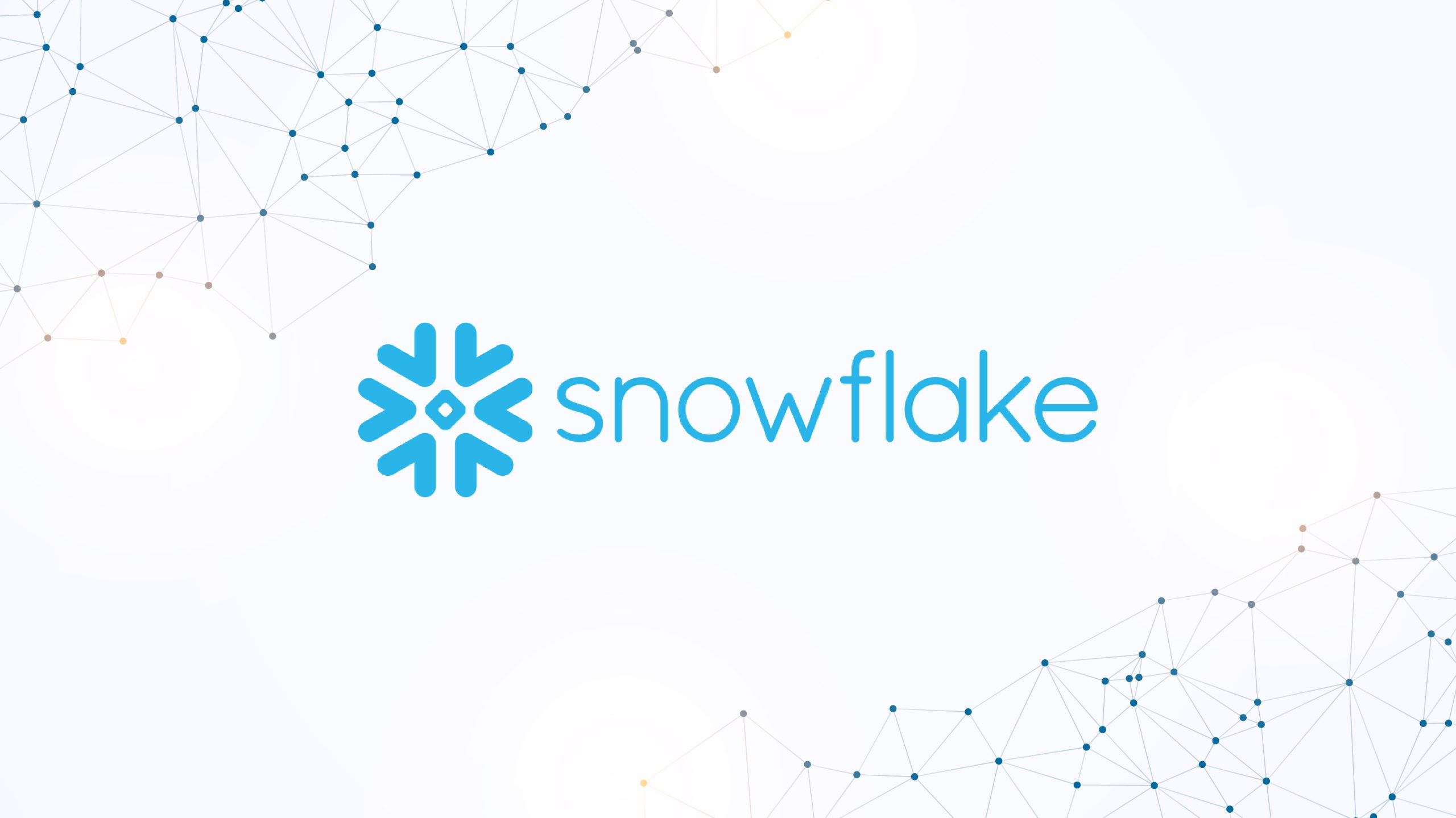
The Want for a Higher Mannequin
Conventional textual content embedding fashions typically include sure limitations together with suboptimal retrieval efficiency, excessive latency, and lack of scalability. These can affect the general person expertise and the practicality of deploying these fashions in real-world enterprise settings.
One of many key challenges with present fashions is their incapacity to constantly ship high-quality retrieval efficiency throughout numerous duties. These embody classification, clustering, pair classification, re-ranking, retrieval, semantic textual similarity, and summarization. Moreover, the dearth of environment friendly sampling methods and competence-aware hard-negative mining can result in subpar high quality within the fashions. Furthermore, the reliance on initialized fashions from different sources could not totally meet the precise wants of enterprises searching for to energy their embedding workflows.
Therefore, there’s a clear want for the event of recent and improved textual content embedding fashions that deal with these challenges. The trade requires fashions that may ship superior retrieval efficiency, decrease latency, and improved scalability. Snowflake’s Arctic embed household of fashions comes as an ideal repair to those limitations. Their give attention to real-world retrieval workloads represents a milestone in offering sensible options for enterprise search and retrieval use instances. Their potential to outperform earlier state-of-the-art fashions throughout all embedding variants additional affirms this.
Past Benchmarks
The Snowflake Arctic embed fashions are particularly designed to empower real-world search functionalities, specializing in retrieval workloads. These fashions have been developed to handle the sensible wants of enterprises searching for to reinforce their search capabilities. By leveraging state-of-the-art analysis and proprietary search information, Snowflake has created a collection of fashions that outperform earlier state-of-the-art fashions throughout all embedding variants. The fashions vary in context window and measurement, with the biggest mannequin standing at 334 million parameters.
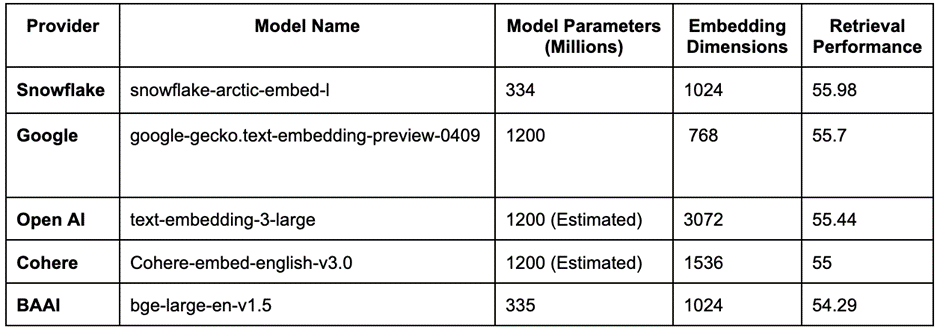
This prolonged context window offers enterprises with a full vary of choices that greatest match their latency, value, and retrieval efficiency necessities. The Snowflake Arctic embed fashions have been evaluated based mostly on the Large Textual content Embedding Benchmark (MTEB). This check measures the efficiency of retrieval programs throughout numerous duties corresponding to classification, clustering, pair classification, re-ranking, retrieval, semantic textual similarity, and summarization. As of April 2024, every of the Snowflake fashions is ranked first amongst embedding fashions of comparable measurement. This demonstrates their unmatched high quality and efficiency for real-world retrieval workloads.
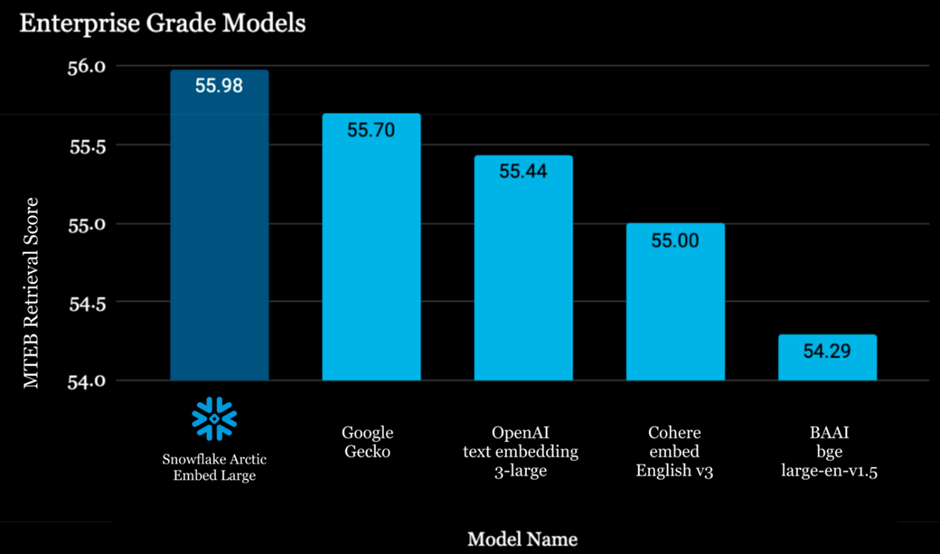
Integration Made Straightforward
The seamless integration of Snowflake Arctic embed fashions with present search stacks is a key function that units these fashions aside. Obtainable immediately from Hugging Face with an Apache 2 license, the fashions could be simply built-in into enterprise search programs with only a few traces of Python code. This ease of integration permits organizations to reinforce their search functionalities with out vital overhead or complexity.
Moreover, the Snowflake Arctic embed fashions have been designed to be extremely straightforward to combine with present search stacks. This offers organizations with a simple and environment friendly course of for incorporating these superior fashions into their search infrastructure. The combination of those fashions with present search stacks allows organizations to leverage their cutting-edge retrieval efficiency whereas seamlessly integrating them into their present search workflows.
Beneath the Hood of Success
The technical superiority of Snowflake’s text-embedding fashions could be attributed to a mixture of efficient strategies from net looking out and state-of-the-art analysis. The fashions leverage improved sampling methods and competence-aware hard-negative mining, leading to large enhancements in high quality. Moreover, Snowflake’s fashions construct on the muse laid by initialized fashions corresponding to bert-base-uncased, nomic-embed-text-v1-unsupervised, e5-large-unsupervised, and sentence-transformers/all-MiniLM-L6-v2. These findings, mixed with net search knowledge and iterative enhancements, have led to the event of state-of-the-art embedding fashions that outperform earlier benchmarks.
A Dedication to the Future
Snowflake is devoted to ongoing improvement and collaboration within the subject of textual content embedding fashions. The discharge of the Snowflake Arctic embed household of fashions is simply step one within the firm’s dedication to offering one of the best fashions for widespread enterprise use instances corresponding to RAG and search.
Leveraging their experience in search derived from the Neeva acquisition, mixed with the info processing energy of Snowflake’s Information Cloud, the corporate goals to quickly develop the kinds of fashions they practice and the focused workloads. Snowflake can also be engaged on growing novel benchmarks to information the event of the subsequent technology of fashions. The corporate encourages collaboration and welcomes strategies from the broader group to additional enhance their fashions.
Conclusion
The Snowflake Arctic embed household of fashions represents a big leap in textual content embedding expertise. By these fashions, Snowflake has achieved state-of-the-art retrieval efficiency, surpassing closed-source fashions with considerably bigger parameters. The potential affect of those fashions lies of their potential to empower real-world retrieval workloads, cut back latency, and decrease the full value of possession for organizations. Their availability in a variety of various sizes and efficiency capabilities reveals Snowflake’s dedication to offering one of the best fashions for widespread enterprise use instances. As we have a good time this launch, the additional improvement of the Arctic embed household is but to be seen.
You’ll be able to discover many extra such AI instruments and their functions right here.