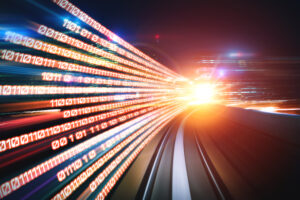
(Summit Artwork Creations/Shutterstock)
Kinetica acquired its begin constructing a GPU-powered database to serve quick SQL queries and visualizations for US authorities and navy shoppers. However with a pair of bulletins at Nvidia’s GTC present final week, the corporate is exhibiting it’s ready for the approaching wave of generative AI functions, significantly these using retrieval augmented technology (RAG) methods to faucet distinctive knowledge sources.
Corporations at present are looking for methods to leverage the facility of huge language fashions (LLMs) with their very own proprietary knowledge. Some corporations are sending their knowledge to OpenAI’s cloud or different cloud-based AI suppliers, whereas others are constructing their very own LLMs.
Nonetheless, many extra corporations are adopting the RAG method, which has surfaced as maybe the perfect center floor between that doesn’t require constructing your individual mannequin (time-consuming and costly) or sending your knowledge to the cloud (not good privateness and security-wise).
With RAG, related knowledge is injected instantly into the context window earlier than being despatched off to the LLM for execution, thereby offering extra personalization and context within the LLMs response. Together with immediate engineering, RAG has emerged as a low-risk and fruitful technique for juicing GenAI returns.
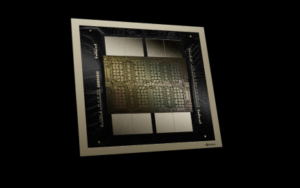
The VRAM increase in Nvidia’s Blackwell GPU will assist Kinetica preserve the processor fed with knowledge, Negahban mentioned
Kinetica can be now stepping into the RAG sport with its database by basically turning it right into a vector database that may retailer and serve vector embeddings to LLMs, in addition to by performing vector similarity search to optimize the information it sends to the LLM.
In keeping with its announcement final week, Kinetica is ready to serve vector embeddings 5x quicker than different databases, a quantity it claims got here from the VectorDBBench benchmark. The corporate claims its capable of obtain that velocity by leveraging Nvidia’s RAPIDS RAFT expertise.
That GPU-based velocity benefit will assist Kinetica prospects by enabling them to scan extra of their knowledge, together with real-time knowledge that has simply been added to the database, with out doing numerous further work, mentioned Nima Negahban, co0founder and CEO of Kinetica.
“It’s onerous for an LLM or a standard RAG stack to have the ability to reply a query about one thing that’s taking place proper now, except they’ve finished numerous pre-planning for particular knowledge sorts,” Negahban advised Datanami on the GTC convention final week, “whereas with Kinetica, we’ll have the opportunity that can assist you by all of the relational knowledge, generate the SQL on the fly, and finally what we put simply again within the context for the LLM is a straightforward textual content payload that the LLM will be capable of perceive to make use of to offer the reply to the query.”
This basically offers customers the potential to speak to their full corpus of relational enterprise knowledge, with out doing any preplanning.
“That’s the massive benefit,” he continued, “as a result of the normal RAG pipelines proper now, that a part of it nonetheless requires a great quantity of labor so far as you need to have the best embedding mannequin, you need to take a look at it, you need to be sure it’s working in your use case.”
Kinetica can even speak to different databases and performance as a generative federated question engine, in addition to do the normal vectorization of knowledge that prospects put within Kinetica, Negahban mentioned. The database is designed for use for operational knowledge, comparable to time-series, telemetry, or teleco knowledge. Due to the help for NVIDIA NeMo Retriever microservices, the corporate is ready to place that knowledge in a RAG workflow.
However for Kinetica, all of it comes again to the GPU. With out the intense computational energy of the GPU, the corporate has simply one other RAG providing.
“Mainly you want that GPU-accelerated engine to make all of it work on the finish of the day, as a result of it’s acquired to have the velocity,” mentioned Negahban, a 2018 Datanami Particular person to Watch. “And we then put all that orchestration on high of it so far as with the ability to have the metadata crucial, with the ability to connect with different databases, having all that to make it straightforward for the tip person, so principally they will begin profiting from all that relational enterprise knowledge of their LLM interplay.”
Associated Objects:
Financial institution Replaces Lots of of Spark Streaming Nodes with Kinetica
Kinetica Goals to Broaden Enchantment of GPU Computing
Stopping the Subsequent 9/11 Aim of NORAD’s New Streaming Information Warehouse