Microsoft is a Chief on this yr’s Gartner® Magic Quadrant™ for Information Science and Machine Studying Platforms. Azure AI gives a robust, versatile end-to-end platform for accelerating information science and machine studying innovation.
Microsoft is a Chief on this yr’s Gartner® Magic Quadrant™ for Information Science and Machine Studying Platforms. Azure AI gives a robust, versatile end-to-end platform for accelerating information science and machine studying innovation whereas offering the enterprise governance that each group wants within the period of AI.
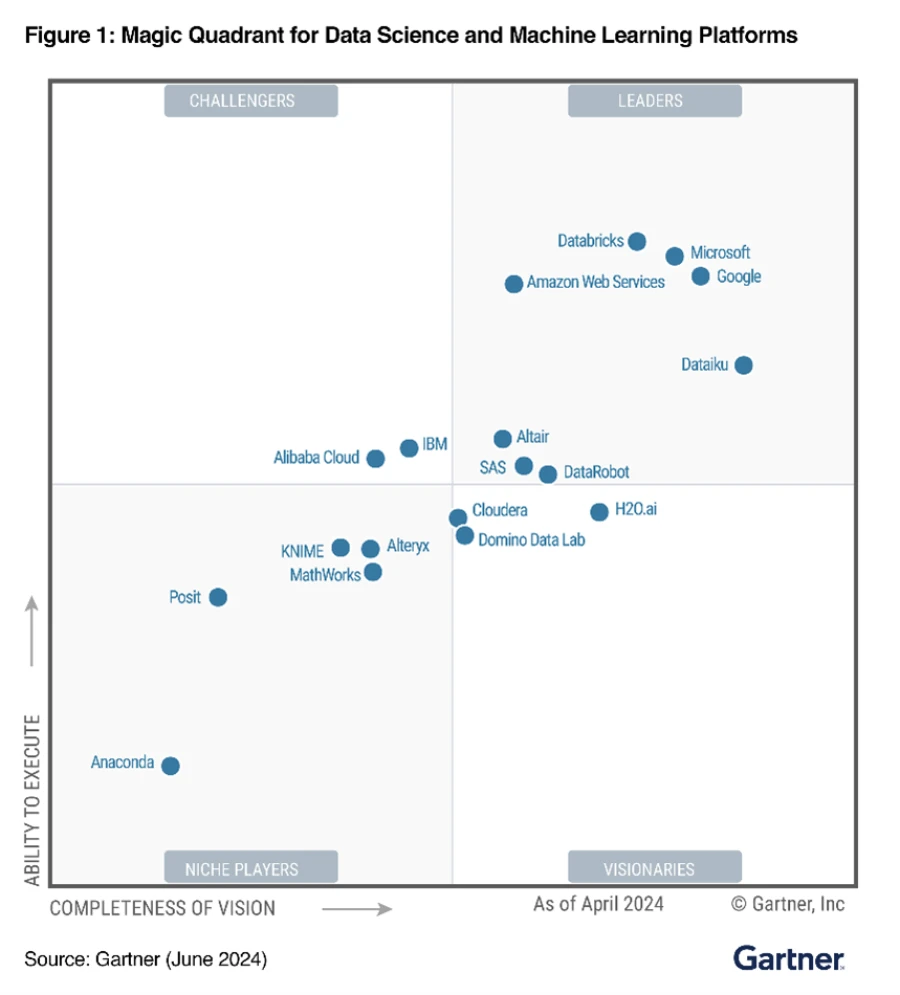
In Might 2024, Microsoft was additionally named a Chief for the fifth yr in a row within the Gartner® Magic Quadrant™ for Cloud AI Developer Providers, the place we positioned furthest for our Completeness of Imaginative and prescient. We’re happy by these recognitions from Gartner as we proceed serving to prospects, from giant enterprises to agile startups, convey their AI and machine studying fashions and purposes into manufacturing securely and at scale.
Azure AI is on the forefront of purpose-built AI infrastructure, accountable AI tooling, and serving to cross-functional groups collaborate successfully utilizing Machine Studying Operations (MLOps) for generative AI and conventional machine studying tasks. Azure Machine Studying gives entry to a broad collection of basis fashions within the Azure AI mannequin catalog—together with the latest releases of Phi-3, JAIS, and GPT-4o—and instruments to fine-tune or construct your personal machine studying fashions. Moreover, the platform helps a wealthy library of open-source frameworks, instruments, and algorithms in order that information science and machine studying groups can innovate in their very own approach, all on a trusted basis.
Speed up time to worth with Azure AI infrastructure
“We’re now in a position to get a functioning mannequin with related insights up and operating in simply a few weeks due to Azure Machine Studying. We’ve even managed to supply verified fashions in simply 4 to 6 weeks.”
Dr. Nico Wintergerst, Employees AI Analysis Engineer at relayr GmbH
Azure Machine Studying helps organizations construct, deploy, and handle high-quality AI options rapidly and effectively, whether or not constructing giant fashions from scratch, operating inference on pre-trained fashions, consuming fashions as a service, or fine-tuning fashions for particular domains. Azure Machine Studying runs on the identical highly effective AI infrastructure that powers a number of the world’s hottest AI companies, reminiscent of ChatGPT, Bing, and Azure OpenAI Service. Moreover, Azure Machine Studying’s compatibility with ONNX Runtime and DeepSpeed might help prospects additional optimize coaching and inference time for efficiency, scalability, and energy effectivity.
Whether or not your group is coaching a deep studying mannequin from scratch utilizing open supply frameworks or bringing an current mannequin into the cloud, Azure Machine Studying allows information science groups to scale out coaching jobs utilizing elastic cloud compute assets and seamlessly transition from coaching to deployment. With managed on-line endpoints, prospects can deploy fashions throughout highly effective CPU and graphics processing unit (GPU) machines with no need to handle the underlying infrastructure—saving effort and time. Equally, prospects don’t have to provision or handle infrastructure when deploying basis fashions as a service from the Azure AI mannequin catalog. This implies prospects can simply deploy and handle hundreds of fashions throughout manufacturing environments—from on-premises to the sting—for batch and real-time predictions.
Streamline operations with versatile MLOps and LLMOps
“Immediate circulate helped streamline our improvement and testing cycles, which established the groundedness we required for ensuring the client and the answer had been interacting in a practical approach.”
Fabon Dzogang, Senior Machine Studying Scientist at ASOS
Machine studying operations (MLOps) and giant language mannequin operations (LLMOps) sit on the intersection of individuals, processes, and platforms. As information science tasks scale and purposes develop into extra advanced, efficient automation and collaboration instruments develop into important for attaining high-quality, repeatable outcomes.
Azure Machine Studying is a versatile MLOps platform, constructed to assist information science groups of any dimension. The platform makes it simple for groups to share and govern machine studying belongings, construct repeatable pipelines utilizing built-in interoperability with Azure DevOps and GitHub Actions, and repeatedly monitor mannequin efficiency in manufacturing. Information connectors with Microsoft sources reminiscent of Microsoft Material and exterior sources reminiscent of Snowflake and Amazon S3, additional simplify MLOps. Interoperability with MLflow additionally makes it seamless for information scientists to scale current workloads from native execution to the cloud and edge, whereas storing all MLflow experiments, run metrics, parameters, and mannequin artifacts in a centralized workspace.
Azure Machine Studying immediate circulate helps streamline your entire improvement cycle for generative AI purposes with its LLMOps capabilities, orchestrating executable flows comprised of fashions, prompts, APIs, Python code, and instruments for vector database lookup and content material filtering. Azure AI immediate circulate can be utilized along with well-liked open-source frameworks like LangChain and Semantic Kernel, enabling builders to convey experimental flows into immediate circulate to scale these experiments and run complete evaluations. Builders can debug, share, and iterate on purposes collaboratively, integrating built-in testing, tracing, and analysis instruments into their CI/CD system to repeatedly reassess the standard and security of their utility. Then, builders can deploy purposes when prepared with one click on and monitor flows for key metrics reminiscent of latency, token utilization, and era high quality in manufacturing. The result’s end-to-end observability and steady enchancment.
Develop extra reliable fashions and apps
“The accountable AI dashboard gives priceless insights into the efficiency and habits of pc imaginative and prescient fashions, offering a greater degree of understanding into why some fashions carry out in a different way than others, and insights into how numerous underlying algorithms or parameters affect efficiency. The profit is better-performing fashions, enabled and optimized with much less effort and time.”
—Teague Maxfield, Senior Supervisor at Constellation Clearsight
AI ideas reminiscent of equity, security, and transparency usually are not self-executing. That’s why Azure Machine Studying gives information scientists and builders with sensible instruments to operationalize accountable AI proper of their circulate of labor, whether or not they should assess and debug a standard machine studying mannequin for bias, defend a basis mannequin from immediate injection assaults, or monitor mannequin accuracy, high quality, and security in manufacturing.
The Accountable AI dashboard helps information scientists assess and debug conventional machine studying fashions for equity, accuracy, and explainability all through the machine studying lifecycle. Customers also can generate a Accountable AI scorecard to doc and share mannequin efficiency particulars with enterprise stakeholders, for extra knowledgeable decision-making. Equally, builders in Azure Machine Studying can assessment mannequin playing cards and benchmarks and carry out their very own evaluations to pick the most effective basis mannequin for his or her use case from the Azure AI mannequin catalog. Then they will apply a defense-in-depth strategy to mitigating AI dangers utilizing built-in capabilities for content material filtering, grounding on recent information, and immediate engineering with security system messages. Analysis instruments in immediate circulate allow builders to iteratively measure, enhance, and doc the influence of their mitigations at scale, utilizing built-in metrics and customized metrics. That approach, information science groups can deploy options with confidence whereas offering transparency for enterprise stakeholders.
Learn extra on Accountable AI with Azure.
Ship enterprise safety, privateness, and compliance
“We would have liked to decide on a platform that offered best-in-class safety and compliance because of the delicate information we require and one which additionally provided best-in-class companies as we didn’t wish to be an infrastructure internet hosting firm. We selected Azure due to its scalability, safety, and the immense assist it presents when it comes to infrastructure administration.”
—Michael Calvin, Chief Technical Officer at Kinectify
In at the moment’s data-driven world, efficient information safety, governance, and privateness require each group to have a complete understanding of their information and AI and machine studying techniques. AI governance additionally requires efficient collaboration between various stakeholders, reminiscent of IT directors, AI and machine studying engineers, information scientists, and threat and compliance roles. Along with enabling enterprise observability by MLOps and LLMOps, Azure Machine Studying helps organizations be certain that information and fashions are protected and compliant with the very best requirements of safety and privateness.
With Azure Machine Studying, IT directors can prohibit entry to assets and operations by consumer account or teams, management incoming and outgoing community communications, encrypt information each in transit and at relaxation, scan for vulnerabilities, and centrally handle and audit configuration insurance policies by Azure Coverage. Information governance groups also can join Azure Machine Studying to Microsoft Purview, in order that metadata on AI belongings—together with fashions, datasets, and jobs—is robotically printed to the Microsoft Purview Information Map. This allows information scientists and information engineers to look at how parts are shared and reused and look at the lineage and transformations of coaching information to grasp the influence of any points in dependencies. Likewise, threat and compliance professionals can monitor what information is used to coach fashions, how base fashions are fine-tuned or prolonged, and the place fashions are employed throughout totally different manufacturing purposes, and use this as proof in compliance studies and audits.
Lastly, with the Azure Machine Studying Kubernetes extension enabled by Azure Arc, organizations can run machine studying workloads on any Kubernetes clusters, guaranteeing information residency, safety, and privateness compliance throughout hybrid public clouds and on-premises environments. This enables organizations to course of information the place it resides, assembly stringent regulatory necessities whereas sustaining flexibility and management over their MLOps. Prospects utilizing federated studying methods together with Azure Machine Studying and Azure confidential computing also can prepare highly effective fashions on disparate information sources, all with out copying or shifting information from safe areas.
Get began with Azure Machine Studying
Machine studying continues to remodel the way in which companies function and compete within the digital period—whether or not you wish to optimize what you are promoting operations, improve buyer experiences, or innovate. Azure Machine Studying gives a robust, versatile machine studying and information science platform to operationalize AI innovation responsibly.
*Gartner, Magic Quadrant for Information Science and Machine Studying Platforms, By Afraz Jaffri, Aura Popa, Peter Krensky, Jim Hare, Raghvender Bhati, Maryam Hassanlou, Tong Zhang, 17 June 2024.
Gartner, Magic Quadrant for Cloud AI Developer Providers, Jim Scheibmeir, Arun Batchu, Mike Fang, Printed 29 April 2024.
GARTNER is a registered trademark and repair mark of Gartner, Inc. and/or its associates within the U.S. and internationally, Magic Quadrant is a registered trademark of Gartner, Inc. and/or its associates and is used herein with permission. All rights reserved.
Gartner doesn’t endorse any vendor, services or products depicted in its analysis publications and doesn’t advise expertise customers to pick solely these distributors with the very best scores or different designation. Gartner analysis publications encompass the opinions of Gartner’s Analysis & Advisory group and shouldn’t be construed as statements of reality. Gartner disclaims all warranties, expressed or implied, with respect to this analysis, together with any warranties of merchantability or health for a selected function.
This graphic was printed by Gartner, Inc. as half of a bigger analysis doc and must be evaluated within the context of your entire doc. The Gartner doc is on the market upon request from this hyperlink.